Unleashing Innovation with Top Use Cases and Cutting-Edge Solutions with Generative AI in Healthcare
Introduction
See Contents
In recent years, the rapid advancement of artificial intelligence (AI) has opened new doors for innovation. This has been across various industries, including the health sector with healthcare software development services. Generative AI, a branch of AI that focuses on creating new content, has emerged as a powerful tool. Mainly with numerous applications in the healthcare sector. From enhancing medical imaging analysis to personalized treatment planning. Generative AI in healthcare offers a multitude of solutions that can significantly benefit healthcare stakeholders.
According to a report by Grand View Research, the market for artificial intelligence in healthcare, which was estimated to be worth USD 15.4 billion in 2022, is anticipated to rise at a CAGR of 37.5% from 2023 to 2030. This indicates the growing adoption of AI technologies, including generative AI, in the healthcare industry.
Also, according to a report by Accenture, the use of AI in healthcare is projected to generate $150 billion in annual savings for the United States healthcare economy by 2026. This emphasizes the significant impact and potential of generative AI in transforming healthcare.
Let us understand generative AI first.
Overview of Generative AI
Generative AI, a subset of artificial intelligence, is dedicated to generating novel content or data. Rather than solely analyzing existing information, it trains models on large datasets. This enables them to learn patterns and structures, which in turn allows them to generate original content similar to the training data.
The Capabilities of Generative AI
Generative AI models have the capability to produce a wide range of outputs, such as images, text, music, and videos. These models find applications in various fields. In fact, it can be including generating art and music, creating content, synthesizing data, and simulating realistic human-like conversations.
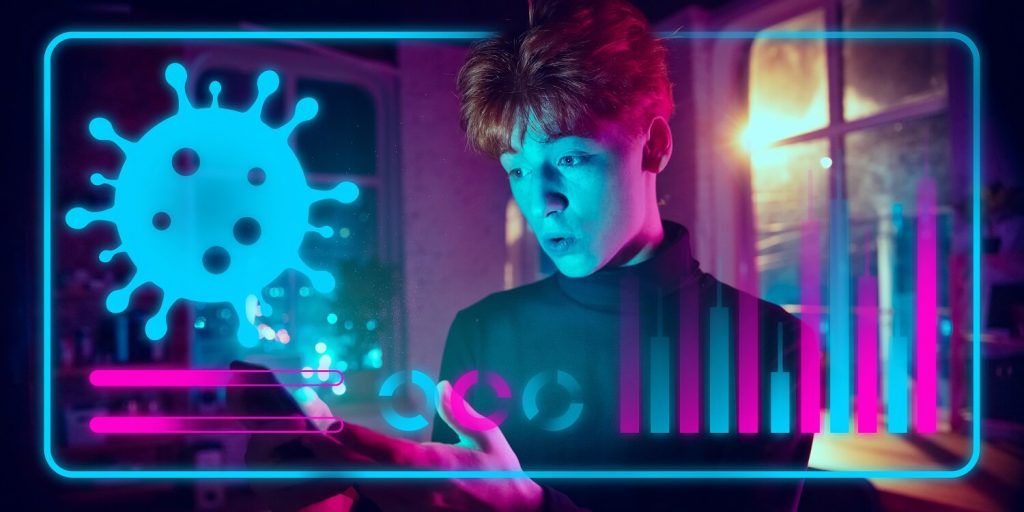
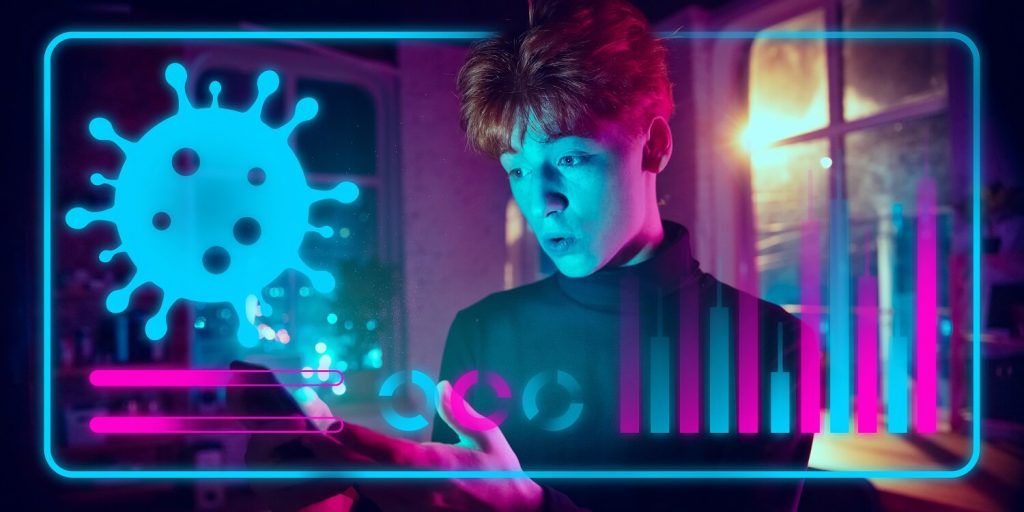
Exploring Generative Adversarial Networks (GANs)
One notable type of generative AI model is the generative adversarial network (GAN). Generative Adversarial Networks (GANs) consist of a generator and a discriminator, working together in a competitive manner. The generator generates new data instances, while the discriminator evaluates the generated data and distinguishes it from real data. This adversarial process enables GANs to learn and generate high-quality, realistic content across various domains, including images, text, and even music. In short, the generator creates new content, like images. While the discriminator distinguishes between the generated content and real examples from the training dataset. Through an iterative process, the generator learns to produce increasingly realistic outputs that can deceive the discriminator.
Advancements in Generative AI
Generative AI has witnessed significant advancements in recent years. As a matter of fact, it has found applications in fields like computer graphics, content creation, and design. Moreover, it holds promise in the healthcare sector. Now, let’s explore some specific use cases of generative AI in healthcare.
Use Cases of Generative AI in Healthcare
I. Enhancing Medical Imaging Analysis
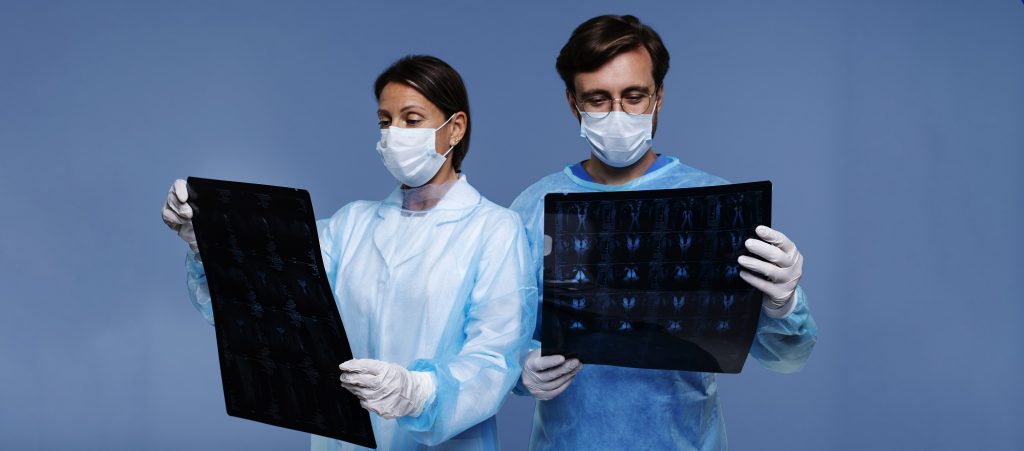
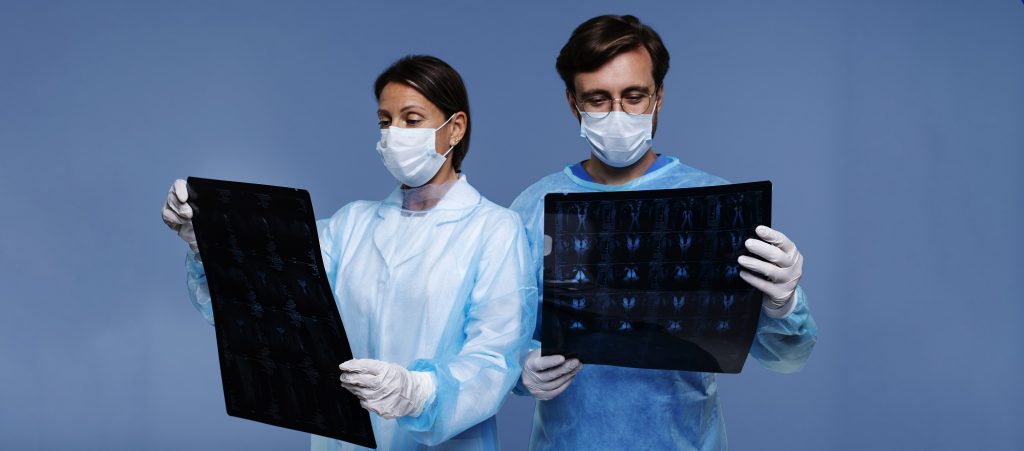
Medical imaging analysis plays a critical role in diagnosing and monitoring diseases. In fact, generative AI solutions offer significant advancements in this field.
Generating high-resolution medical images
Generative adversarial networks (GANs) can generate high-resolution medical images with fine details. Generative AI can be employed to generate high-resolution medical images, aiding in improving image quality and also enhancing details. This can be especially useful in areas such as radiology and pathology. This is where clear and accurate imaging is very crucial for accurate diagnoses.
For example, a study published in Nature Communications demonstrated the use of GANs to generate high-resolution brain MRI images, which helped improve the accuracy of brain tumor segmentation.
Assisting in radiology and pathology diagnoses
Generative AI models can assist radiologists and pathologists in analyzing medical images. In fact, by leveraging deep learning algorithms, these models can highlight potential abnormalities or assist in identifying specific features. Moreover, thereby supports more accurate and efficient diagnoses.
Generative AI models, such as convolutional neural networks (CNNs), can assist radiologists and pathologists in diagnosing diseases. A study published in Nature Medicine journal showed that a CNN model outperformed human radiologists in detecting breast cancer in mammograms.
Predicting disease progression from imaging data
Generative AI techniques can analyze longitudinal medical imaging data to predict disease progression. By detecting subtle changes in images over time, these models can provide insights into disease trajectories, also helping healthcare professionals make informed decisions regarding treatment plans and interventions.
A study published in Pubmed used GANs to predict Alzheimer’s disease progression from brain MRI scans with high accuracy.
II. Generating Synthetic Patient Data for Research
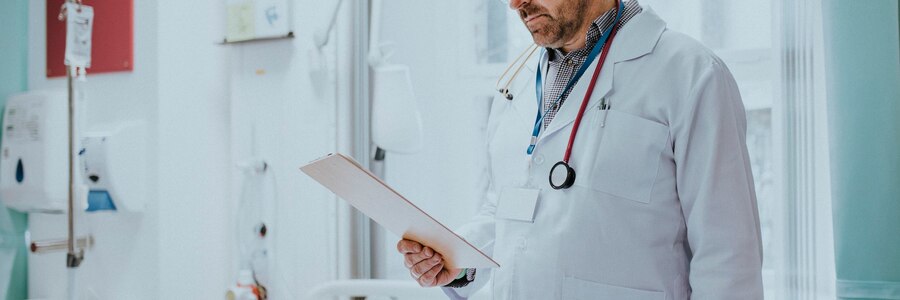
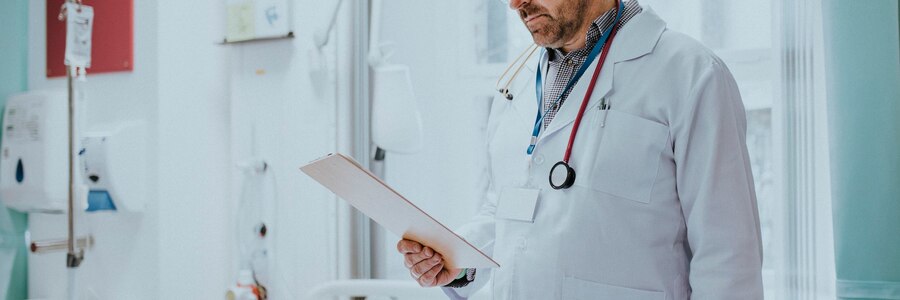
Research in healthcare often requires access to large and diverse datasets. Generative AI can generate synthetic patient data, offering valuable resources for various research purposes.
Creating synthetic patient records for data augmentation
Generative AI techniques can generate synthetic patient records, which can be used to augment real-world patient data for research. This expands the dataset size, leading to more comprehensive analyses and potentially uncovering new insights.
A study published in Nature Digital Medicine demonstrated the use of generative models to create synthetic electronic health records for research purposes. EHR-M-GAN has proven to be superior to state-of-the-art benchmarks. It is for synthesizing clinical time series with high fidelity. While addressing the data types and also dimensionality restrictions in the existing generative models.
Simulating diverse patient populations for clinical trials
Generative AI models can simulate diverse patient populations, enabling researchers to conduct virtual clinical trials. This helps optimize trial designs, evaluate treatment effectiveness, and enhance the generalizability of trial results.
A study published in JAMA Network Open utilized generative models to simulate diverse patient populations for cardiovascular disease clinical trials.
Enabling privacy-preserving data sharing for research collaborations
Generative AI techniques, such as federated learning, enable privacy-preserving data sharing among healthcare institutions. This allows researchers to collaborate and train models collectively without directly sharing sensitive patient information, ensuring compliance with privacy regulations.
A study published in Pubmed highlighted the potential of federated learning for multi-center studies while preserving patient privacy.
III. Personalized Treatment Planning
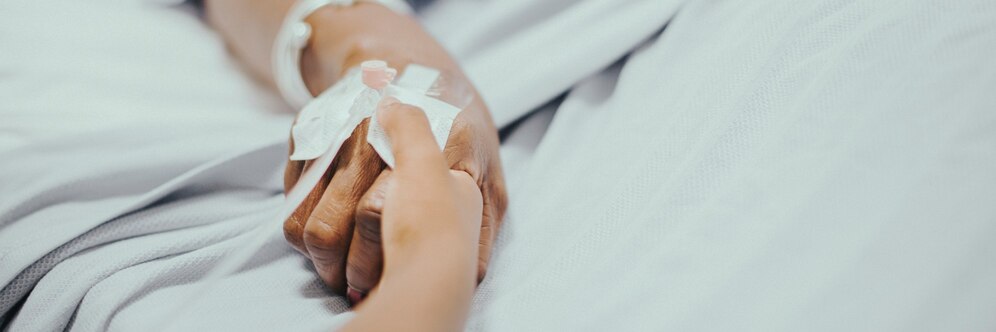
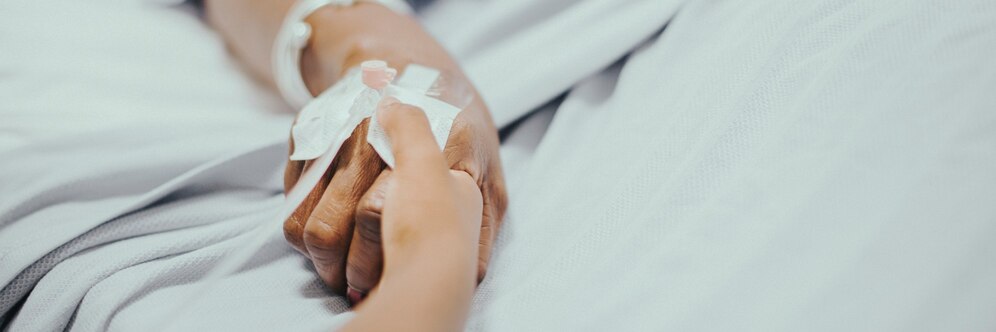
Personalized treatment planning aims to tailor healthcare interventions based on individual patient characteristics. Generative AI solutions offer opportunities for precise and optimized treatment strategies.
Creating patient-specific treatment plans based on medical history and genetics
Generative AI models can analyze patient data. In fact, it includes medical history, genetic information, and other relevant factors, to develop personalized treatment plans. This accounts for individual variations and also optimizes treatment strategies, leading to more effective and targeted healthcare interventions.
A study published in The Lancet Oncology demonstrated the use of generative models. This was to create patient-specific treatment plans for cancer patients.
Optimizing drug dosages and treatment schedules for individual patients
Generative AI algorithms can analyze patient data and drug response information to optimize drug dosages and treatment schedules. This helps avoid under or over-treatment and maximizes therapeutic outcomes.
A study published in NCBI reports utilized generative models to optimize drug dosages for patients with Parkinson’s disease.
Assisting in surgical planning and precision medicine
Generative AI techniques, such as 3D modeling and virtual simulations, can aid in surgical planning and precision medicine. By analyzing patient-specific data and simulating surgical procedures. Healthcare professionals can better understand complex anatomical structures, optimize surgical approaches, and also improve patient outcomes.
A study published in the NCBI showcased the use of generative models for surgical planning in craniofacial surgeries.
IV. Drug Discovery and Development
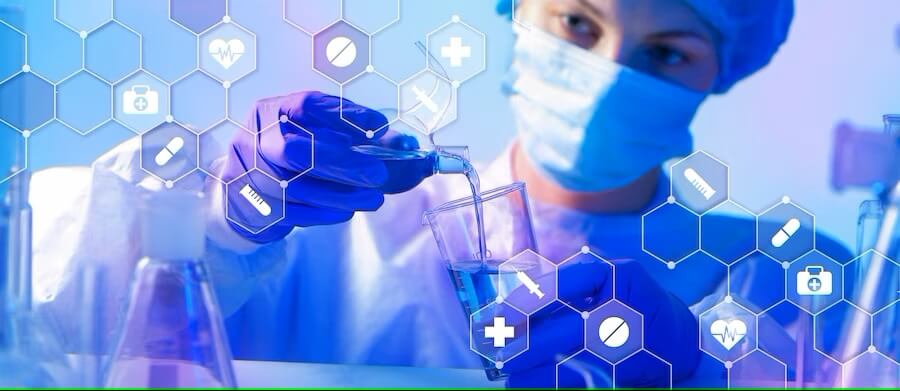
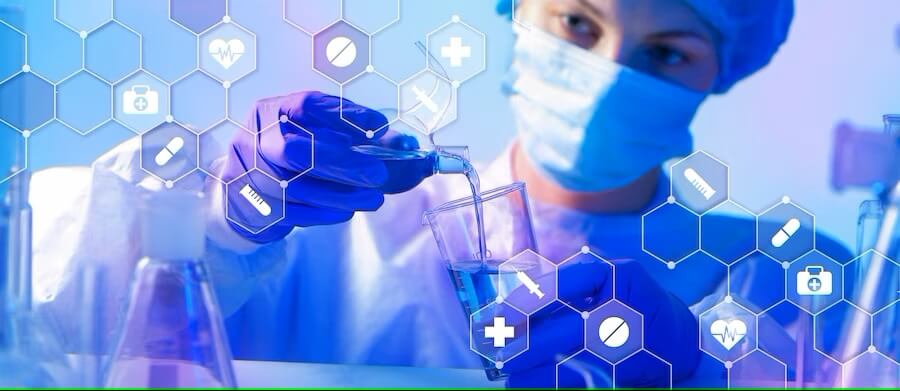
The use of generative AI in drug discovery and development has gained momentum in recent years.
Designing Novel Molecules and Compounds for Drug Discovery
Generative AI can accelerate the drug discovery process by designing novel molecules and compounds with desired properties. By exploring vast chemical spaces, these models can generate potential drug candidates, saving time and resources in the initial stages of drug development.
A study published in the Nature Journal demonstrated the success of generative AI in designing novel molecules with desired properties. According to MIT researches the AI-generated molecule, named Halicin, showed promising antibacterial activity against drug-resistant strains.
Accelerating Virtual Screening and Lead Optimization
Generative AI techniques can aid in virtual screening, allowing researchers to quickly identify promising lead compounds with high binding affinity to specific targets. This accelerates the identification of potential drug candidates, streamlining the lead optimization process in drug development.
A research article published in the journal Science Direct highlighted the role of generative AI in accelerating virtual screening and lead optimization. The study utilized generative models to identify potent drug candidates for various diseases, reducing the time and cost associated with traditional screening methods.
Predicting Drug-Target Interactions and Side Effects
Generative AI models can predict drug-target interactions and potential side effects by analyzing molecular structures, biological pathways, and existing knowledge. This helps prioritize and identify drug candidates with favorable target interactions and reduced risks of adverse effects, facilitating safer and more efficient drug development.
Generative AI has shown promise in predicting drug-target interactions and potential side effects. A study published in the journal PLOS Computational Biology demonstrated the use of generative models to predict drug-protein interactions, facilitating the identification of new drug targets and minimizing the risk of adverse effects.
V. Medical Report Generation
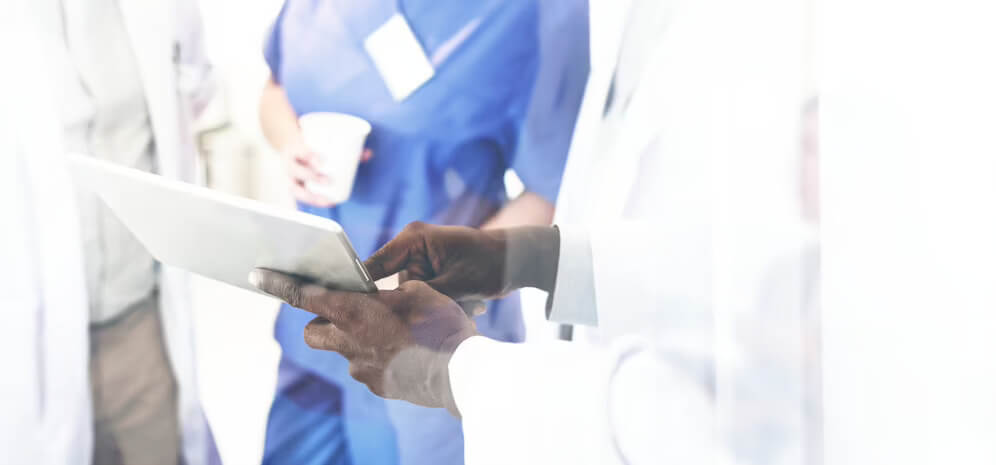
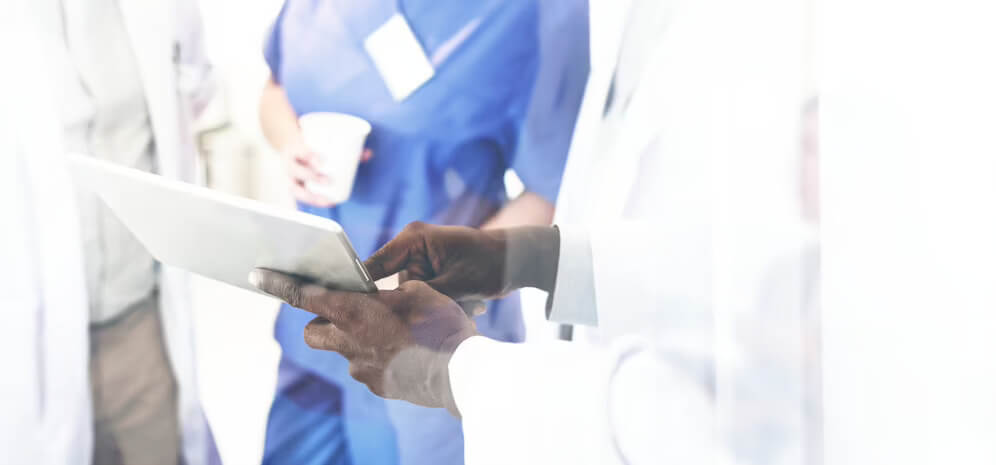
Generative AI has the potential to revolutionize the process of medical report generation, benefiting both healthcare providers and patients.
Automatically Generating Structured Medical Reports from Patient Data
Generative AI algorithms can analyze patient data, including clinical notes, imaging results, and laboratory reports, to automatically generate structured medical reports. This streamlines the documentation process, reduces clinician workload, and ensures consistent and comprehensive reporting.
A research paper published in the NCBI states Artificial Intelligence in Medicine proposed a generative AI framework for automatically generating structured medical reports. The study demonstrated that the generated reports were comparable in quality to reports produced by human experts.
Summarizing Patient Conditions, Diagnoses, and Treatment Recommendations
Generative AI models can extract key information from patient data and generate concise summaries of patient conditions, diagnoses, and treatment recommendations. These summaries provide a quick overview for healthcare professionals, aiding in decision-making and facilitating efficient communication among care teams.
A study published in the journal JAMA Network Open showcased the use of natural language processing techniques to generate accurate and comprehensive summaries from electronic health records.
Assisting in Efficient Documentation and Reducing Clinician Workload
By automating the generation of medical reports, generative AI reduces the time and effort required for documentation, allowing healthcare professionals to focus more on direct patient care. This not only improves workflow efficiency but also contributes to better patient outcomes.
VI. Augmenting Telemedicine and Remote Patient Monitoring
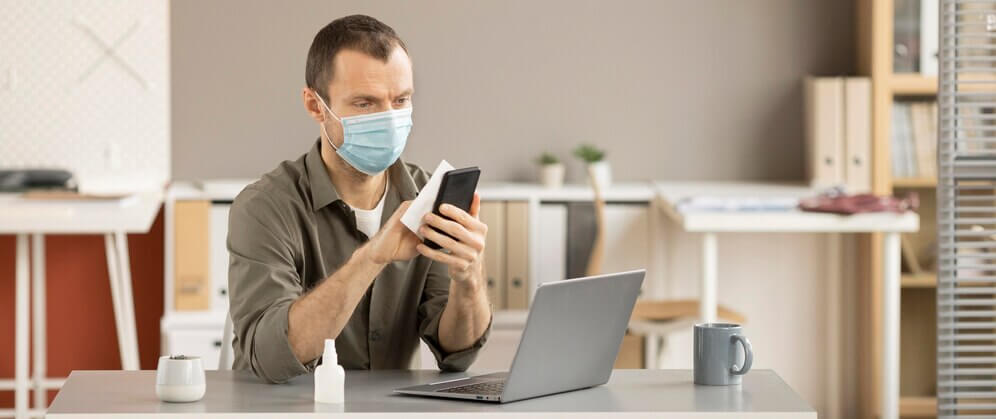
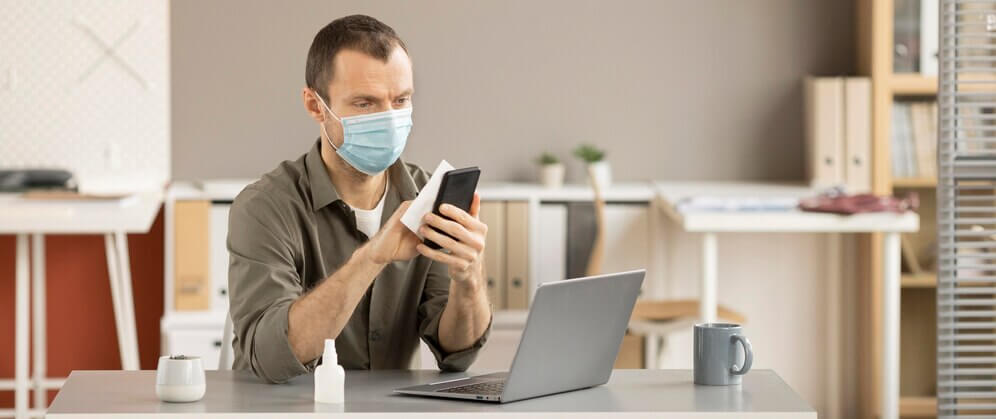
Generative AI plays a crucial role in augmenting telemedicine and remote patient monitoring, especially in the era of remote healthcare delivery.
Enabling Remote Monitoring of Vital Signs and Health Indicators
Generative AI, combined with wearable devices and sensors, can enable remote monitoring of patient’s vital signs and health indicators. Real-time data analysis and anomaly detection algorithms can provide early warnings for potential health issues, allowing timely interventions and remote healthcare delivery.
A research article published in Nature demonstrated the use of generative AI in the remote monitoring of vital signs. The study utilized AI algorithms to analyze data from wearable devices and predict health indicators, enabling early detection of health issues.
Supporting Virtual Consultations with Realistic Patient Avatars
Generative AI models can generate realistic patient avatars that simulate various medical conditions, facilitating virtual consultations. These avatars can help healthcare providers visualize and communicate diagnoses and treatment options effectively, even in remote settings.
Generative AI models can generate realistic patient avatars that simulate various medical conditions. A study published in the journal JMIR Medical Education highlighted the potential of virtual patient avatars in supporting virtual consultations and medical education.
Enhancing Tele-Diagnosis and Remote Healthcare Delivery
Generative AI can support tele-diagnosis by analyzing patient data, medical images, and symptoms. By providing decision support and assisting in remote diagnostics, generative AI enhances the capabilities of healthcare professionals, particularly in areas with limited access to specialized care.
Click to read more detail on Telemedicine App Development Solutions
VII. Improving Disease Diagnosis
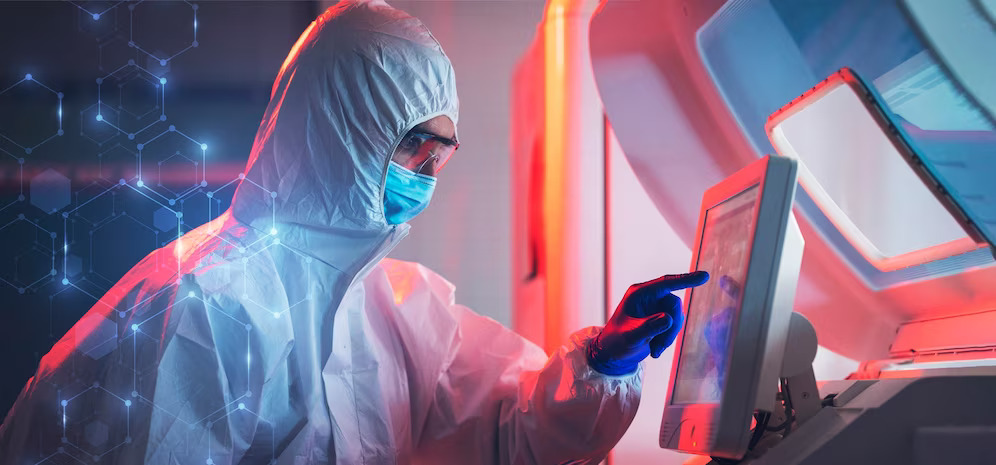
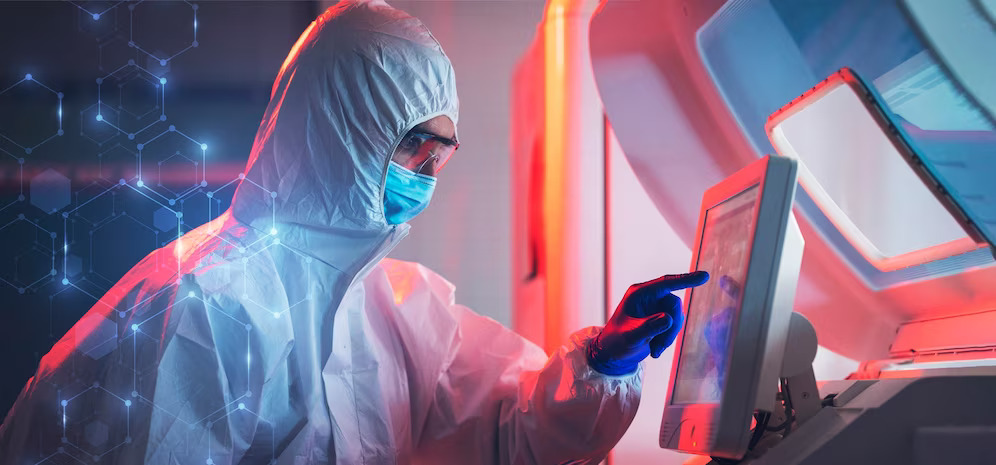
Generative AI has the potential to revolutionize disease diagnosis by providing advanced decision support and analysis capabilities.
Assisting in the Early Detection and Diagnosis of Diseases
Generative AI models can analyze various patient data, including medical images, laboratory results, and genetic profiles, to aid in the early detection and diagnosis of diseases. By recognizing subtle patterns and indicators, these models support healthcare providers in making accurate and timely diagnoses.
A study published in NCBI demonstrated the effectiveness of generative AI in detecting skin cancer with high accuracy, comparable to that of dermatologists.
Predicting Disease Outcomes and Progression
Generative AI can analyze longitudinal patient data to predict disease outcomes and progression. By integrating multi-modal data and utilizing predictive modeling techniques, these models offer insights into disease trajectories, enabling proactive interventions and personalized treatment plans.
A study published in Pubmed utilized generative models to predict the progression of chronic kidney disease, aiding in personalized treatment planning and interventions.
Identifying Patterns and Biomarkers for Improved Diagnostic Accuracy
Generative AI algorithms can identify patterns and biomarkers within complex datasets, contributing to improved diagnostic accuracy. By uncovering hidden relationships and markers that are difficult for humans to detect, these models enhance the understanding and classification of various diseases.
VIII. Virtual Patient Simulations
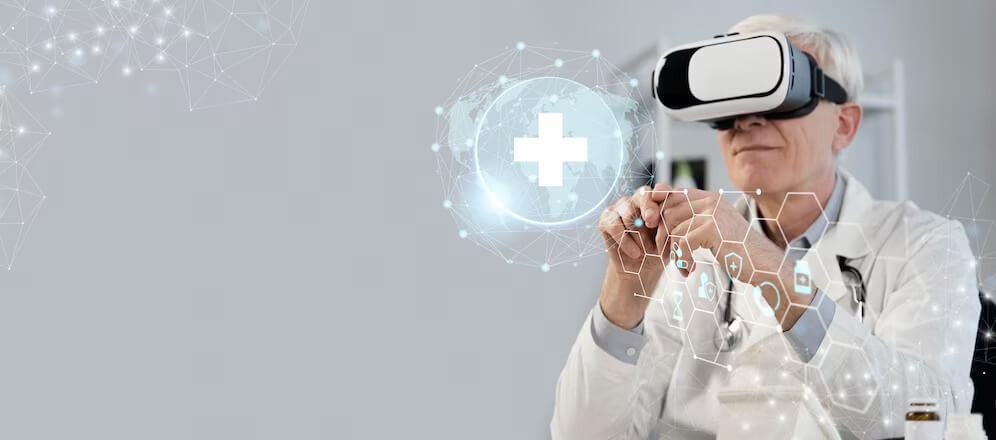
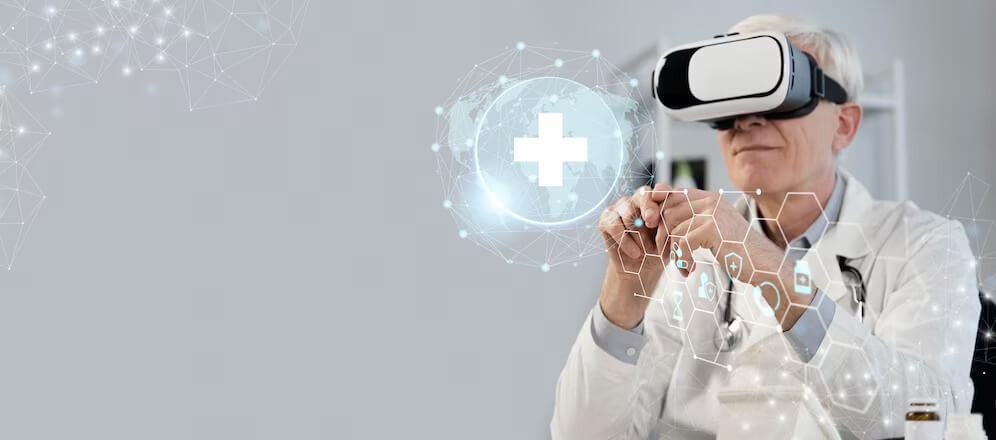
Creating Virtual Patient Models for Medical Education and Training
Generative AI has revolutionized medical education and training by creating virtual patient models that mimic real-world cases. These virtual patients offer realistic and interactive learning experiences for healthcare professionals, allowing them to practice clinical skills, decision-making, and surgical procedures in a risk-free environment.
A study published in NCBI found that virtual patient simulations improved medical students’ knowledge retention and clinical reasoning skills.
Simulating Realistic Clinical Scenarios for Healthcare Professionals
Generative AI models can simulate realistic clinical scenarios, providing healthcare professionals with opportunities to develop and refine their diagnostic and treatment skills. These simulations offer virtual environments that mirror real-world challenges, allowing healthcare professionals to enhance their competence and readiness in managing complex medical cases.
A study published in NCBI reported that surgical simulations improved technical skills and decision-making abilities among surgical residents.
Enhancing Surgical Simulations and Procedure Planning
Generative AI techniques have significantly enhanced surgical simulations and procedure planning. Surgeons can use these simulations to virtually practice complex procedures, evaluate different approaches, and anticipate potential challenges. By optimizing surgical planning, generative AI improves surgical outcomes and patient safety.
A study published in NCBI demonstrated that surgical simulations reduced operating room time and improved surgical precision.
IX. Health Monitoring and Predictive Analytics
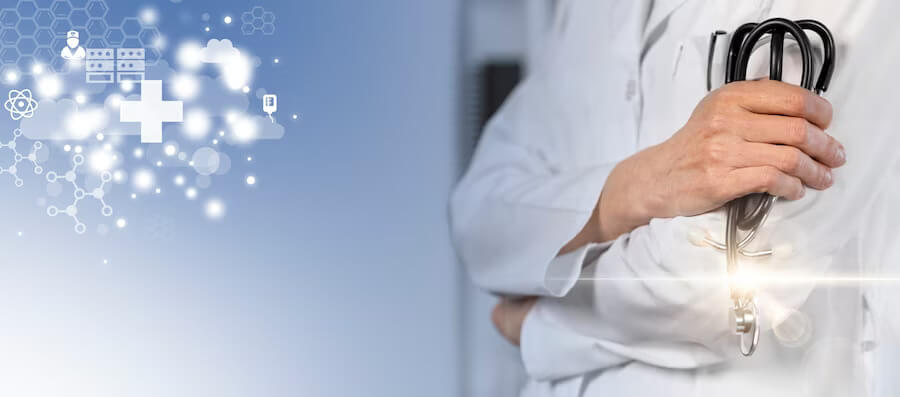
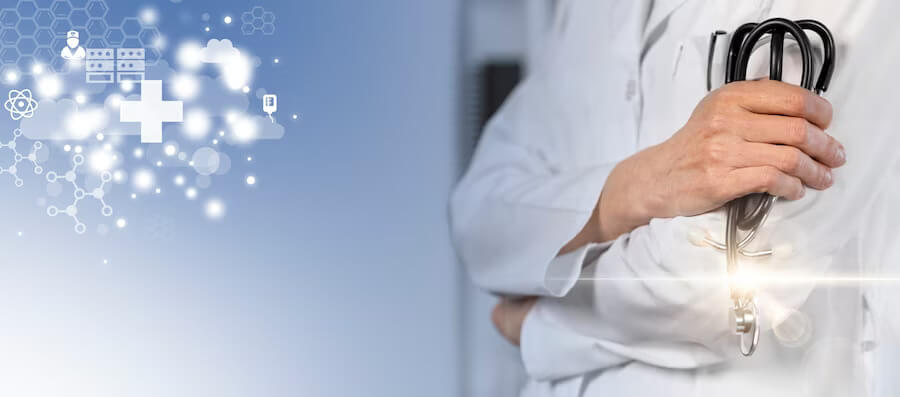
Analyzing Sensor Data for Real
Time Health Monitoring: Generative AI models can analyze sensor data from wearable devices, remote monitoring systems, and electronic health records to provide real-time health monitoring. These models detect anomalies, trends, and risk indicators, enabling proactive interventions and timely healthcare interventions.
A study published in NCBI demonstrated the effectiveness of generative AI in analyzing sensor data to detect early signs of deterioration in patients with chronic conditions.
Predicting Disease Outbreaks and Public Health Trends
Generative AI epidemiological data and predictive analytics can forecast disease outbreaks and identify public health trends. By analyzing large-scale data, including demographic information, environmental factors, and social media data, generative AI models assist in early detection and prevention strategies.
For example, a study published in NCBI used wearable devices and generative AI to predict influenza outbreaks with high accuracy, enabling timely public health interventions.
Identifying At-Risk Populations for Proactive Interventions
Generative AI analyzes diverse healthcare data sources to identify at-risk populations for various health conditions. This information helps healthcare providers target interventions, allocate resources efficiently, and implement preventive measures to improve population health outcomes.
A study published in the Journal of the American Medical Informatics Association utilized generative AI to identify populations at risk of developing type 2 diabetes, facilitating targeted preventive interventions.
X. Mental Health Support and Therapy
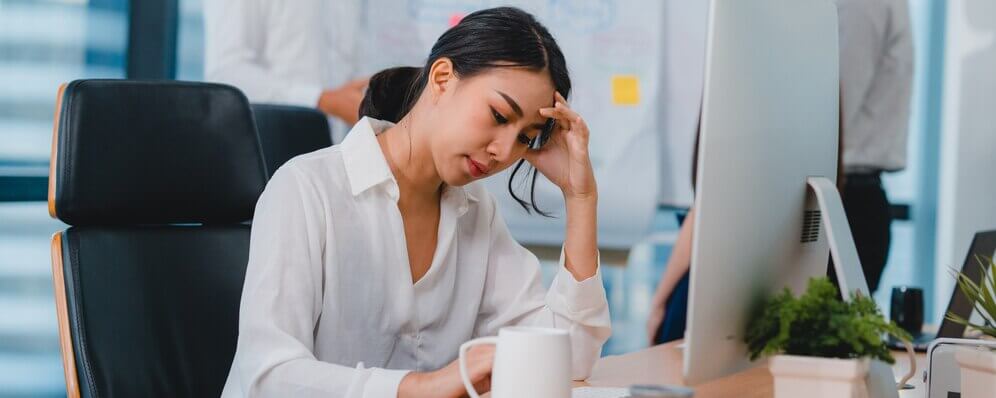
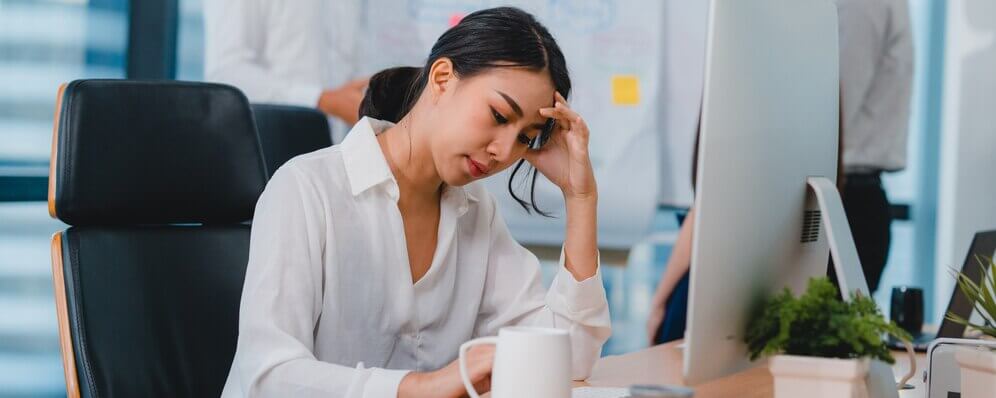
Developing Virtual Therapists for Mental Health Counseling
Generative AI has paved the way for virtual therapists or chatbots that provide mental health counseling. These AI-powered conversational agents offer emotional support, and coping strategies, and engage in therapeutic dialogues, complementing traditional mental health services.
A study published in Frontiers Media explored using a generative AI chatbot for mental health support and found that it improved psychological well-being among users.
Generating Interactive and Empathetic Chatbots for Emotional Support
Generative AI models can generate interactive and empathetic chatbots that simulate human-like conversations. These chatbots provide a safe space for individuals to express their emotions, receive guidance, and access mental health resources.
A study published in the journal Frontiers in Psychology demonstrated the effectiveness of generative AI chatbots in reducing symptoms of depression and anxiety among users.
Personalizing Therapeutic Interventions Based on Individual Needs
Generative AI algorithms analyze individual characteristics, behavior patterns, and treatment response data to personalize therapeutic interventions. By tailoring interventions to specific needs, generative AI enhances the effectiveness of mental health treatments and supports individual well-being.
A study published in Pubmed highlighted the potential of generative AI in personalizing cognitive-behavioral therapy for individuals with depression.
These applications of generative AI in healthcare demonstrate its wide-ranging potential to transform various aspects of the industry. However, it is essential to continue research and development while considering ethical considerations, data privacy, and regulatory guidelines to ensure the responsible and beneficial use of generative AI technologies.
Challenges With Generative AI in Healthcare
Generative AI in healthcare faces several challenges. One significant concern is the ethical implications of generating synthetic medical data that could potentially be misleading or used maliciously. Ensuring the privacy and security of patient information is paramount, as generative models trained on sensitive data could inadvertently reveal personally identifiable information.
Another challenge is the need for robust validation and regulation of generative AI models to ensure their safety, reliability, and effectiveness in real-world healthcare settings. Additionally, the interpretability of generative AI outputs poses a challenge, as it can be difficult to understand and explain the reasoning behind the generated content, leading to potential trust issues among healthcare professionals.
Overcoming these challenges requires collaboration between AI researchers, healthcare providers, ethicists, and regulatory bodies to establish clear guidelines, standards, and safeguards for the responsible deployment of generative AI in healthcare.
What are the strategies to overcome challenges in using generative AI in healthcare?
To overcome the challenges associated with generative AI in healthcare, several approaches can be taken:
1. Robust Data Privacy and Security
Implement strong data anonymization techniques to protect patient privacy. Adhere to strict security protocols to safeguard sensitive healthcare data from unauthorized access or breaches. Comply with relevant regulations such as HIPAA (Health Insurance Portability and Accountability Act) to ensure patient data protection.
How can compliance with generative AI be maintained in the healthcare industry?
To maintain compliance with the use of generative AI in healthcare, organizations should adhere to relevant regulations and standards, such as HIPAA, GDPR, and local data protection laws. They should implement robust data privacy and security measures, including anonymization techniques and strict access controls. Regular audits and assessments can ensure compliance with industry guidelines. Collaboration with regulatory bodies, policymakers, and legal experts can help stay updated on evolving compliance requirements. Transparency in model development, validation, and evaluation processes is crucial. Additionally, organizations should promote a culture of ethical awareness, training, and accountability to ensure responsible and compliant use of generative AI in healthcare.
Click to check more details on healthcare compliance.
2. Rigorous Validation and Regulation
Establish standardized validation procedures and guidelines for generative AI models in healthcare. Encourage transparent reporting of model development, training methodologies, and evaluation metrics. Regulatory bodies can play a crucial role in reviewing and approving generative AI applications to ensure patient safety and efficacy.
3. Explainability and Interpretability
Develop techniques to enhance the interpretability of generative AI outputs. Researchers can explore methods to provide explanations for the generated content, such as attention mechanisms or visualization techniques. Promote the use of interpretable architectures and techniques to build trust and enable effective collaboration between AI systems and healthcare professionals.
4. Ethical Frameworks and Guidelines
Foster interdisciplinary collaboration involving AI researchers, healthcare professionals, ethicists, and policymakers to develop ethical frameworks and guidelines specifically tailored to generative AI in healthcare. These frameworks should address issues such as bias, fairness, accountability, and informed consent to mitigate potential ethical concerns.
5. Robust Model Evaluation
Establish standardized evaluation metrics and benchmarks for generative AI models in healthcare. Rigorously evaluate the performance, safety, and generalizability of these models before deployment. Encourage transparency in reporting model limitations, potential risks, and uncertainties associated with generated outputs.
6. Collaborative Partnerships
Foster collaborations between AI researchers, healthcare institutions, and regulatory bodies to ensure that generative AI technologies are developed and implemented responsibly. Encourage open dialogue, knowledge sharing, and cooperation to address the challenges collectively.
7. Continuous Monitoring and Improvement
Implement mechanisms for continuous monitoring and improvement of generative AI models in healthcare. Regularly update and retrain models to adapt to evolving medical knowledge, changes in patient demographics, and emerging ethical considerations. Encourage feedback loops from healthcare professionals and patients to refine and optimize generative AI systems.
By implementing these strategies and approaches, it is possible to navigate the challenges associated with generative AI in healthcare and pave the way for responsible and beneficial use of these technologies in improving patient care and outcomes.
Conclusion
Generative AI has paved the way for groundbreaking advancements in healthcare, transforming how stakeholders tackle challenges and deliver care. From enhancing medical imaging analysis to personalized treatment planning, the use cases of generative AI in healthcare are vast and promising. With solutions such as synthetic patient data generation, drug discovery acceleration, and virtual patient simulations, healthcare professionals and researchers can leverage generative AI to improve patient outcomes, optimize treatment strategies, and advance medical knowledge.
As generative AI continues to evolve, it is crucial to ensure ethical considerations such as patient privacy, bias mitigation, and transparency. Collaboration between AI experts, healthcare product engineering partner, healthcare professionals, and policymakers is vital to harness the full potential of generative AI in healthcare. By embracing these technologies responsibly, we can usher in a new era of patient-centric care, innovation, and improved health outcomes for all.
Connect with us for exploring generative AI solutions.
2 Replies to “Top Use Cases and Cutting-Edge Solutions with Generative AI in Healthcare”
Comments are closed.